Том 334 № 12 (2023)
DOI https://doi.org/10.18799/24131830/2023/12/4407
Прогнозирование электропотребления с помощью нейронных сетей c LSTM
Актуальность работы обусловлена необходимостью улучшения точности прогнозирования электропотребления для повышения его эффективности и, как следствие, для улучшения конкурентоспособности производимой продукции за счёт сокращения доли затрат на оплату электроэнергии в ее себестоимости. При определении прогнозных показателей потребления электроэнергии промышленными предприятиями с высокой точностью целесообразно использовать современные апробированные методы прогнозирования. Из существующих в настоящее время приблизительно 150 методов прогнозирования только 20–30 активно используются на практике. Анализ существующих методов прогнозирования, применяемых на промышленных предприятиях, показывает, что они преимущественно основаны либо на экспертной оценке объёмов электропотребления, либо на учёте удельного расхода электроэнергии (на единицу производимой продукции). Цель: повысить точность прогнозирования электропотребления промышленными предприятиями с использованием метода искусственного интеллекта, в частности метода искусственной нейронной сети, в том числе метода Long-short Time Memory (LSTM). Методы: При разработке модели прогнозирования были использованы методы искусственной нейронной сети, в том числе метод Long-short Time Memory (LSTM). При обработке первичных данных были использованы методы нормального закона распределение Гаусса, нормирование/масштабирование. Результаты подтверждены расчетным путем с применением предлагаемой модели на основе метода искусственной нейронной сети для прогнозирования электропотребления промышленных предприятий. Важное достоинство метода – наличие возможности его обучения и адаптирования к процессу прогнозирования. Реальными расчетами показано, что их удается успешно завершить за счет правильного подбора состава входящих слоёв и исключения случайностей.
Ключевые слова:
прогнозирование, электропотребление, ошибка прогнозирования, адекватность модели, однослойная нейронная сеть, функция активации, нейроны, обучение, тестирование, валидация, алгоритм, ошибка, входной слой, весовые коэффициенты, среднеквадратичная ошибка
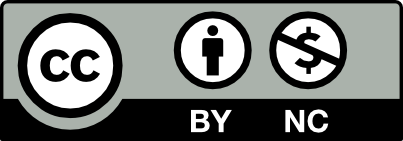