Том 334 № 12 (2023)
DOI https://doi.org/10.18799/24131830/2023/12/4253
Прогнозирование почасовой выработки электроэнергии солнечной электростанцией с помощью алгоритмов машинного обучения
Актуальность исследования заключается в необходимости развития энергосберегающих подходов за счет применения средств интеллектуального анализа данных для повышения эффективности процесса принятия управленческих решений и, как следствие, более оптимального использования энергетических ресурсов. В частности, прогнозирование величины выработки электрической энергии солнечной электростанцией позволит повысить обоснованность решений, принимаемых в процессе управления распределением электроэнергии в децентрализованных системах. При наличии информации о том, сколько электроэнергии будет выработано солнечной электростанцией и передано в сеть в каждый час суток, появится возможность более обоснованно планировать использование выработанной электроэнергии и ее распределение. Также наличие достоверного прогноза позволит встраивать математическую модель в подсистему управления микросетями, что, в свою очередь, будет способствовать интеграции централизованных электрических сетей и объектов распределенной генерации. На практике для реализации прогнозирования не всегда подходят существующие методы расчета выработки электроэнергии солнечными электростанциями по техническим характеристикам оборудования солнечных электростанций. Кроме того, нет стандартов по использованию метеорологических и других факторов при создании прогнозных моделей. Поэтому исследование носит междисциплинарный характер, сочетая в себе важную практическую значимость и выработку новых рекомендаций в части применения алгоритмов машинного обучения, а также учета метеорологических факторов в прогнозных моделях. Целью исследования является повышение обоснованности перспективного планирования объемов выработки электрической энергии объектом альтернативной энергетики (солнечной электростанцией). Объекты: солнечная электростанция им А.А. Влазнева (Сакмарская СЭС), расположенная в Оренбургской области, г. Орск, Октябрьский район, географические координаты: 51.266023 с.ш, 58.474689 з.д. Методы: аналитический метод, методы математической статистики, методы машинного обучения, комплексного обобщения научных достижений и практического опыта применения средств обработки данных в задачах прогнозирования выработки электроэнергии солнечными электростанциями. Результаты. Проведен обзор литературных источников, отражающих результаты применения современных средств интеллектуального анализа данных при прогнозировании величины выработки электроэнергии солнечными электростанциями. Рассмотрены различные подходы к прогнозированию выработки электроэнергии на солнечных электростанциях, проведен анализ факторов, применяемых при прогнозировании. Были получены результаты теоретического и прикладного характера, заключающиеся в рекомендациях по применению экзогенных переменных при прогнозировании выработки электроэнергии на СЭС, а также некоторых алгоритмов машинного обучения при построении прогнозных моделей. Данные рекомендации были получены в процессе обобщения результатов прикладных исследований.
Ключевые слова:
Солнечная энергия, энергосбережение, прогнозирование, интеллектуальный анализ данных, машинное обучение, градиентный бустинг
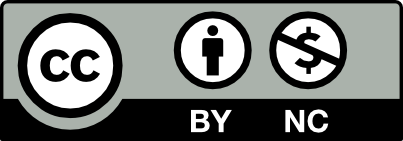